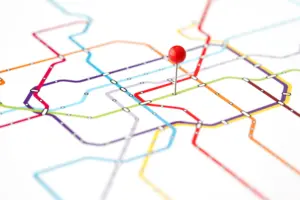
Cracking the Churn Code: Finding Where Churn Originates
For too long, the actual steps of analysing churn has been kept a trade secret. Not anymore. We believe in transparency and knowledge sharing, so we’re opening our books to share our approach to help you Crack the Churn Code.