Cracking the Churn Code: Finding Where Churn Originates
The term “churn” often looms large, carrying profound implications for customer relations and profitability. While many discuss its significance, the practical methods to decipher the origins of churn often remain shrouded in secrecy.
At Vaxa Analytics, we believe in transparency and knowledge sharing. In this instalment of our series, we peel back the curtain and unveil our precise methodology for understanding churn dynamics. From deciphering complex graphs to delving into insightful cohort analyses, we guide you step-by-step.
With our approach, demystifying churn becomes not just a theoretical exercise but a tangible, actionable process. We illuminate the path to discern where changes can be made, allowing you to cater to your customers’ needs effectively and precisely when they need it most.
This blog post is part of our Cracking the Churn Code series, part of our Demystifying Analytics program. See our full series here, and keep an eye out as we explore this topic in great detail – but this time, in plain English.
Tracking Churn Over Time: Unraveling Trends
When it comes to understanding any customer behaviour and indeed, business performance, time is the most crucial dimension.
By tracking churn rates over various periods—ranging from monthly to quarterly to yearly—we gain valuable insights into the macro nature of the relationship between your business and your customers.

However, the effectiveness of this analysis heavily depends on the precision and adaptability of the metrics used, as well as the underlying data quality. It’s vital to have a well-calculated metric that can seamlessly adapt to different time periods – there are a lot of definitions to calculate “churn” out there, but we have one that we think is the most robust.
Long-term trends provide a panoramic view of customer loyalty and engagement. By identifying these patterns, such as a gradual increase in churn over several quarters, businesses can strategise for sustainable growth. Sudden fluctuations, on the other hand, demand immediate attention. They could signify external factors like market shifts or internal issues such as service disruptions or customer dissatisfaction.
Don’t forget – even the time of the month can significantly impact churn rates. For instance, customers might be more likely to churn around payment dates or their pay slips.
Time of year also plays a big part—good old-fashioned seasonality.
Consider a gym. By tracking monthly churn rates, the business might notice a spike in cancellations during the winter months — when getting out of bed early becomes harder. This insight could lead to a targeted effort to offer more after-work/weekend classes, bring-a-friend-for-free, or special “pay upfront and save” (aka “lock yourself in”) options. We’ll explore how you might track the effectiveness of this later in the piece.

In another scenario, a corporate-focused SaaS company might observe increased churn around the end of the financial year when budgets are reassessed. Recognising this pattern allows the company to proactively engage with clients, offering tailored solutions or discounts to incentivise renewals well before the year-end budget cuts take effect. Even better if they use other data they have collected (e.g. product analytics) to better demonstrate and reiterate the value their solution provides.

Understanding these time-based patterns helps you anticipate and prepare for fluctuations, enabling proactive retention efforts ahead of these critical periods.
But how do we understand the impact of our retention efforts?
Cohort Analysis: Understanding Where To Play And The Impact of Changes
Cohort analysis allows us to dissect customer data based on specific characteristics or time frames. In most cases, you’ll develop cohorts based on the sign-up date.
By scrutinising these cohorts, your business can gain profound insights into customer behaviour when it comes to their subscriptions; as cohorts are groups of customers who share common traits or experiences within a particular time frame, the analysis speaks directly to the experience of alike customers within those cohorts.
Here’s an example cohort analysis. We’re going to keep on the topic of subscriptions, but the exact same approach can be used for ad-hoc purchases too.
In this case, we’ve created cohorts based on the sign-up date on the Y-axis.

On the X-axis, you’ll note we don’t use calendar months, we use months relative to their signup date. So, 1 month after signup, 2 months after signup. That’s critical to how we use cohort analysis.
Finding Your Key Hurdles
Examining our cohorts “vertically” — picking e.g. Month 3 or Month 4 from the X-axis—we can uncover how our customers perceive value over time. Is month 3 when they start to regret their decision to sign up? Or is it actually Month 6? Can you think of some strategies to address churn—before it happens—at these critical points?

We can also often see the classic bathtub curve in these charts; churn is often higher in the first few months of a subscription, then will stabilise as the remaining subscribers are more committed.
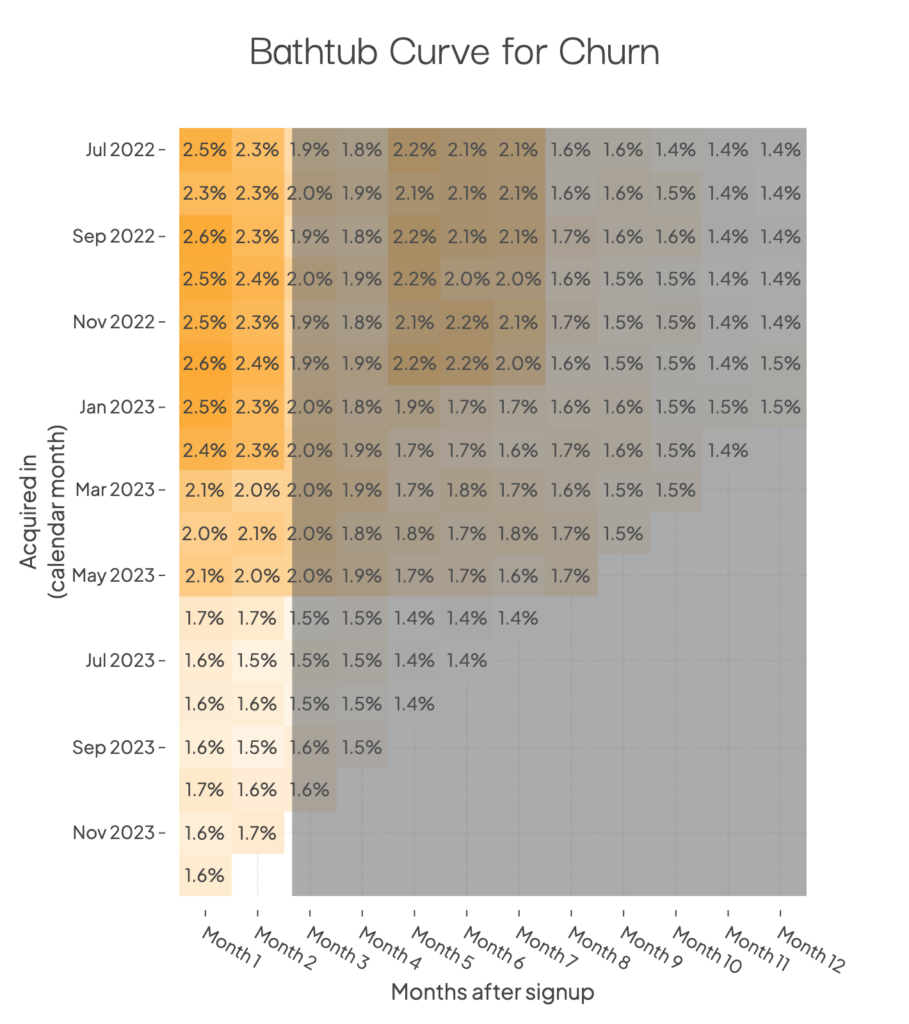
Changing Acquisition Strategies
Lots of subscription businesses will change tact and alter how/where they’re finding customers—often for a good reason. But as we know, acquisition is only half the challenge. Are these “different” subscribers sticking around long enough to make this strategy stack up? That is to say, are we finding the right type of new subscriber?
Cohort analysis can do that, too.

By comparing May with June (when this hypothetical new acquisition strategy started), we can see that yes, our strategy does in fact find subscribers who stick around for long enough. Great!
Tracking Your Impact
You can also use cohorts to understand the real-world impact of your efforts. Let’s say in Jan 2023 we introduced a hyper-targeted retention program for our subscribers reaching the 6-month mark (the hurdle from earlier). Did it make a difference?

Well, we can see that our cohorts who are reaching the 6-month mark after we made this intervention are sticking around for a lot longer — meaning our efforts are working!
The Gold Standard: A/B Testing
Coupled with this standard cohort analysis, A/B testing (where possible) will provide you with a gold-standard method to gauge the impact of your efforts in addressing customer churn.
By comparing two groups—one exposed to a change (e.g., a new pricing tier) and the other kept unchanged—you can quantitatively measure the effect on churn rates, no ifs or buts. This empirical approach offers invaluable insights, enabling data-driven decision-making for effective—both in effort and in cost—churn management strategies.
Cohort analysis, paired with the precision of A/B testing, equips your business with a robust toolkit to not only comprehend the impact of changes but also proactively enhance customer experiences, thereby reducing churn rates and fostering long-term customer relationships.
Our favourite, approachable and free resource for learning all about this approach is The Open Guide to Successful AB Testing by our friends over at Growthbook – a platform we love and use for our client’s AB testing efforts. It’s well worth the read.

Segmentation Insights: Cracking Churn in Customer Groups
Segmentation is the key to so many powerful insights – and customer churn is no exception. By categorising customers into distinct segments based on various criteria such as demographics, behaviour, or preferences, we can conduct targeted analyses that offer deeper insights into customer behaviour.
Understanding how different segments respond to changes not only refines the analysis but also guides our strategy for actions to take, as we can theorise how different segments will respond to different treatments.
Overlaying Segments: Enhancing Sensitivity in Analysis
By simply conducting the exact same analysis we presented above, but just filtered to each of your segments, your analysis gains a whole layer of nuanced depth. It’s particularly powerful when analysing churn.
Treating all customers as a homogeneous group can lead to oversights. Customer behaviours and reasons for churning can vary significantly across different segments. For instance, low-value (low monthly $ value) customers might churn for different reasons than long-term or high-value customers – they’re likely more price-sensitive. By dissecting the customer base into segments, businesses can pinpoint the specific factors influencing churn within each group.
Indeed, in some cases, your overall churn may not exhibit any of the trends we just reviewed above – but by overlaying your segments, this suddenly appears.
That’s why we say overlaying different segments amplifies the sensitivity of churn analysis.
Overlaying different segments amplifies the sensitivity of churn analysis.
By examining churn rates within each segment, businesses can uncover hidden patterns and trends that might remain obscured in broader analyses.
For example, a slight increase in the overall churn rate might not raise concerns, but if this increase is concentrated within a high-value customer segment, it becomes a critical issue that demands immediate attention.
A segment consisting of customers who primarily engage through mobile apps might show different churn behaviours compared to those who use desktop platforms – both due to how they were acquired, but also the experience they have using this different platform.
Linking Segmentation and AB Testing
So we’ve established some customer segments and identified churn that’s specific to some segments and not others.
We start to theorise why Segment X churns more than Segment Y. But how will we know?
To truly understand this, we must back up our segmentation insights with A/B testing. This way we can craft targeted retention strategies that address the unique needs of each segment, and be confident that our efforts are truly driving the result (and not random chance).
This sort of segment-specific A/B testing requires a solid data management framework to ensure you can target the right segment, record which variant each customer is in, and then record the outcomes. We’d be happy to help.
Consider Segment X, comprising price-sensitive customers, and Segment Y, consisting of customers seeking premium services. We’ve built these segments based on a combination of survey data during onboarding, external data enrichment services, and the price plan they selected.
We theorise Segment X responds positively to discounts and special offers, while Segment Y values personalised experiences and exclusive access to premium features. We also theorise that by highlighting these angles to each respective segment, we’ll see a reduction in churn.
A/B testing confirms these insights: Segment X shows increased engagement and reduced churn when offered discounts, whereas Segment Y responds better to personalised loyalty programs and early access to new features.
When our efforts align seamlessly with customer segments, it creates a win-win situation.
Your customers feel valued and understood, leading to increased loyalty and reduced churn. Moreover, your business experiences higher ROI on its retention efforts, as resources are directed precisely where they yield the most impact. This symbiotic relationship between segmentation analysis and A/B testing results in a dynamic, responsive churn management strategy that adapts to the evolving needs of different customer segments.
Navigating The Future: In Conclusion
Understanding churn isn’t just about observing patterns—it’s about deciphering the language of your customers, spoken through their actions and choices. By exploring the intricacies of time-based trends, delving into cohort analyses, and overlaying different customer segments, you gain the power to predict, respond, and ultimately, retain.
The synergy between segmentation analysis and A/B testing presents an opportunity for your business to refine its strategies, reaching customers in the most meaningful ways.
As you embark on your journey to crack the churn code, remember: every churned customer represents not just a loss but an opportunity. An opportunity to learn, adapt, and create an experience so exceptional that it becomes a stronghold against churn’s relentless tide.
Armed with insights, strategies, and an unwavering commitment to customer satisfaction, your business is now better poised to navigate the future with confidence, ensuring lasting relationships and enduring success.